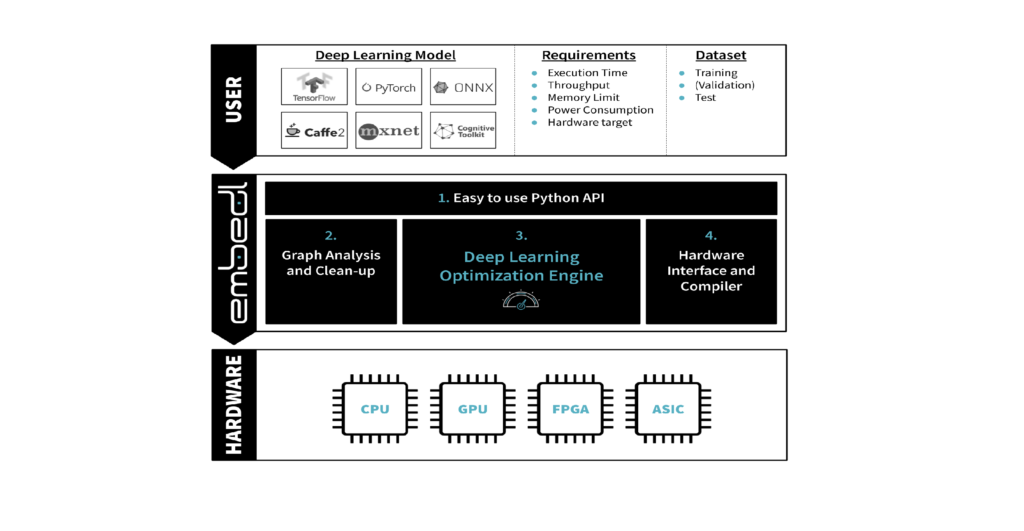
In the world of embedded artificial intelligence (AI), the race is on to achieve high-performance computing while minimizing energy consumption. Swedish startup Embedl is at the forefront of this endeavor, offering a deep learning optimization engine to reduce execution time, improve performance, and lower power consumption when deploying AI on embedded devices. Their groundbreaking approach has recently attracted significant funding, totaling SEK 45 million (over $4 million), to expand their operations, particularly in the automotive industry.
The Power of Optimization
Embedl’s core proposition is straightforward yet revolutionary: simplify computational models and reduce execution times to achieve lower energy consumption, higher throughput, and increased accuracy. The company employs cutting-edge techniques such as hardware-aware pruning, quantization, and neural architecture search (NAS) to significantly reduce execution time for deep neural networks on embedded hardware. Pruning and quantization reduce the number of computations needed for each network layer, while NAS identifies the most efficient network architecture for a specific application.
As the demand for AI systems continues to grow across industries, energy efficiency is paramount. Embedl’s approach aims to reduce energy consumption at the system level, thus contributing positively to the environment. Their solution has the potential to greatly enhance performance in the development of autonomous driving systems and advanced driving assistance systems (ADAS) within the automotive sector. It also allows AI to be integrated into consumer products with less powerful hardware. Additionally, it enables deep learning algorithms to run efficiently on battery-powered devices, avoiding excessive energy consumption.
The Benefits of Reduced Execution Time
Reducing execution time offers multiple advantages. Faster inference speeds are a key benefit, enabling data to be processed more rapidly and leading to real-time responses with increased accuracy. This is especially crucial in applications like autonomous vehicles, where swift decision-making is vital for safety and efficiency.
Embedl’s offerings are already making an impact in the market. Founded in 2018 as a spinout from the European Horizon 2020 funded research project LEGato, the startup boasts a scalable product with a growing customer base, including leading AI companies. Their customers rely on Embedl to enhance the performance of their end products, expedite time-to-market, and reduce hardware costs.
Scaling Up with New Funding
Embedl’s recent investment round, led by Spintop Ventures with co-financing from the European Innovation Council (EIC), and participation from S-E Bankens Utvecklingsstiftelse, angel investors, key employees, and existing investors, is a testament to the potential of their technology. This funding will further empower Embedl to focus on their core value proposition and expand their horizons. Ola Tiverman, Embedl’s Chief Product Officer, stated that they plan to hire more researchers, engineers, and other professionals, enhance their hardware-aware optimization of AI models for edge devices, expand their product portfolio, and take their solutions internationally.
Embedl’s journey is an exciting one, demonstrating that innovative deep learning model optimization can be a game-changer for embedded AI. As they continue to grow and refine their technology, the impact on various industries, from automotive to consumer electronics, is sure to be significant.
Leave a comment